On Friday, a team of researchers at the University of Chicago released a research paper outlining “Nightshade,” a data poisoning technique aimed at disrupting the training process for AI models, reports MIT Technology Review and VentureBeat. The goal is to help visual artists and publishers protect their work from being used to train generative AI image synthesis models, such as Midjourney, DALL-E 3, and Stable Diffusion.
The open source “poison pill” tool (as the University of Chicago’s press department calls it) alters images in ways invisible to the human eye that can corrupt an AI model’s training process. Many image synthesis models, with notable exceptions of those from Adobe and Getty Images, largely use data sets of images scraped from the web without artist permission, which includes copyrighted material. (OpenAI licenses some of its DALL-E training images from Shutterstock.)
AI researchers’ reliance on commandeered data scraped from the web, which is seen as ethically fraught by many, has also been key to the recent explosion in generative AI capability. It took an entire Internet of images with annotations (through captions, alt text, and metadata) created by millions of people to create a data set with enough variety to create Stable Diffusion, for example. It would be impractical to hire people to annotate hundreds of millions of images from the standpoint of both cost and time. Those with access to existing large image databases (such as Getty and Shutterstock) are at an advantage when using licensed training data.
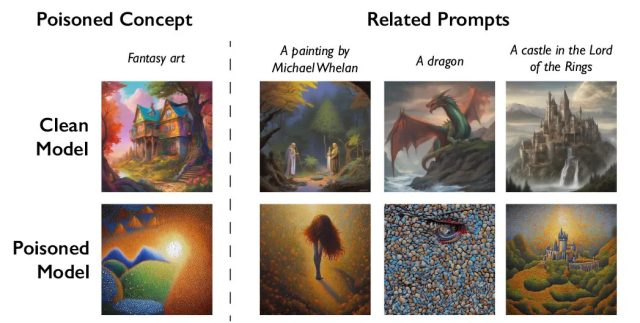
Shan, et al.
Along those lines, some research institutions, like the University of California Berkeley Library, have argued for preserving data scraping as fair use in AI training for research and education purposes. The practice has not been definitively ruled on by US courts yet, and regulators are currently seeking comment for potential legislation that might affect it one way or the other. But as the Nightshade team sees it, research use and commercial use are two entirely different things, and they hope their technology can force AI training companies to license image data sets, respect crawler restrictions, and conform to opt-out requests.
“The point of this tool is to balance the playing field between model trainers and content creators,” co-author and University of Chicago professor Ben Y. Zhao said in a statement. “Right now model trainers have 100 percent of the power. The only tools that can slow down crawlers are opt-out lists and do-not-crawl directives, all of which are optional and rely on the conscience of AI companies, and of course none of it is verifiable or enforceable and companies can say one thing and do another with impunity. This tool would be the first to allow content owners to push back in a meaningful way against unauthorized model training.”
Shawn Shan, Wenxin Ding, Josephine Passananti, Haitao Zheng, and Zhao developed Nightshade as part of the Department of Computer Science at the University of Chicago. The new tool builds upon the team’s prior work with Glaze, another tool designed to alter digital artwork in a manner that confuses AI. While Glaze is oriented toward obfuscating the style of the artwork, Nightshade goes a step further by corrupting the training data. Essentially, it tricks AI models into misidentifying objects within the images.
For example, in tests, researchers used the tool to alter images of dogs in a way that led an AI model to generate a cat when prompted to produce a dog. To do this, Nightshade takes an image of the intended concept (e.g., an actual image of a “dog”) and subtly modifies the image so that it retains its original appearance but is influenced in latent (encoded) space by an entirely different concept (e.g., “cat”). This way, to a human or simple automated check, the image and the text seem aligned. But in the model’s latent space, the image has characteristics of both the original and the poison concept, which leads the model astray when trained on the data.