The US Math Olympiad (USAMO) serves as a qualifier for the International Math Olympiad and presents a much higher bar than tests like the American Invitational Mathematics Examination (AIME). While AIME problems are difficult, they require integer answers. USAMO demands contestants write out complete mathematical proofs, scored for correctness, completeness, and clarity over nine hours and two days.
The researchers evaluated several AI reasoning models on the six problems from the 2025 USAMO shortly after their release, minimizing any chance the problems were part of the models’ training data. These models included Qwen’s QwQ-32B, DeepSeek R1, Google’s Gemini 2.0 Flash Thinking (Experimental) and Gemini 2.5 Pro, OpenAI’s o1-pro and o3-mini-high, Anthropic’s Claude 3.7 Sonnet with Extended Thinking, and xAI’s Grok 3.
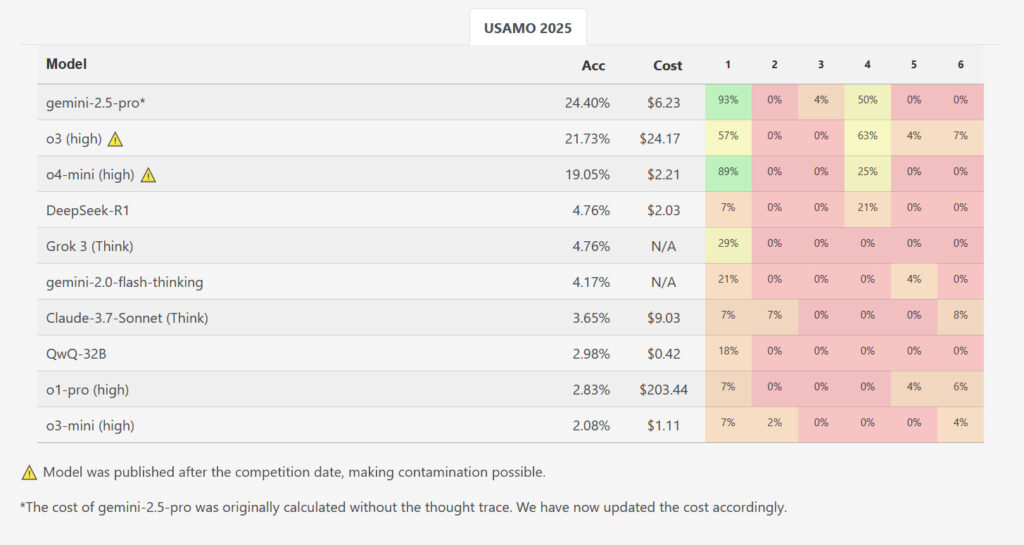
While one model, Google’s Gemini 2.5 Pro, achieved a higher average score of 10.1 out of 42 points (~24 percent), the results otherwise showed a massive performance drop compared to AIME-level benchmarks. The other evaluated models lagged considerably further behind: DeepSeek R1 and Grok 3 averaged 2.0 points each, Google’s Flash-Thinking scored 1.8, Anthropic’s Claude 3.7 managed 1.5, while Qwen’s QwQ and OpenAI’s o1-pro both averaged 1.2 points. OpenAI’s o3-mini had the lowest average score at just 0.9 points (~2.1 percent). Out of nearly 200 generated solutions across all tested models and runs, not a single one received a perfect score for any problem.
While OpenAI’s newly released 03 and o4-mini-high were not examined for this study, benchmarks at the researchers’ MathArena website show o3-high scoring 21.73 percent overall and o4-mini-high scoring 19.05 percent overall on USAMO. However, those results are potentially contaminated because they were measured after the contest took place, meaning that the newer OpenAI models could potentially have included the solutions in the training data.
How the models failed
In the paper, the researchers identified several key recurring failure patterns. The AI outputs contained logical gaps where mathematical justification was lacking, included arguments based on unproven assumptions, and continued producing incorrect approaches despite generating contradictory results.
A specific example involved USAMO 2025 Problem 5. This problem asked models to find all positive whole numbers “k,” such that a specific calculation involving sums of binomial coefficients raised to the power of “k” would always result in an integer, no matter which positive integer “n” was used. On this problem, Qwen’s QwQ model made a notable error: It incorrectly excluded non-integer possibilities at a stage where the problem statement allowed them. This mistake led the model to an incorrect final answer despite having correctly identified the necessary conditions earlier in its reasoning process.